Coventry University CRISP PhD Student!
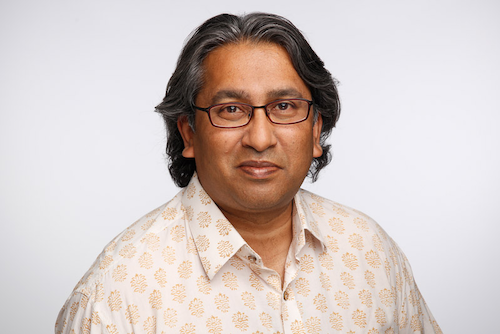
Was Rahman is a PhD student at Coventry University, under the supervision of Professors Sally Dibb and Maureen Meadows. His PhD investigates AI fairness in business decision-making.
Was has a long-standing professional and academic interest in how we enable, assure and govern ethical use of technologies, especially those that evolve more quickly than laws and regulations controlling them. This interest builds on his experience of working with AI and data technologies for large businesses and government departments since the 1990s. A central principle of his research is that understanding “fairness” is crucial to building “fairer AI”
News reports of “unfair” AI are all too familiar, stretching back beyond Amazon’s inadvertently sexist recruitment system and Google’s facial recognition system confusing black humans with gorillas. However, addressing this unfairness in challenging, not least because different academic disciplines have different views not just on how to address such issues, but also on what the actual issue is to address. Indeed, the concept of fairness is often treated superficially in existing work.
Was is exploring the implications of disciplinary differences in understanding AI fairness for research, business and regulatory/policy audiences. His work highlights the diverse definitions and approaches used being used work across academic disciplines, including computing, law, management, politics, psychology and neuroscience.
Early findings suggest that many researchers and business practitioners typically approach their work with a relatively narrow, discipline-specific view about what fairness means. For example, computer science tends to treat AI unfairness in explicit – quantified – mathematical terms, whereas social scientists often take a more qualitative approach, focusing on implicit attitudes and behaviours rooted in socio-economic and colonial history. Meanwhile, legal scholars may emphasise demonstrable, material harm linked to specific protected characteristics.
A specific focus for the research is on discrimination towards under-represented groups in business decision-making. Using interview data and publicly available information, the research critically reviews the literature and of common AI fairness assurance approaches and mechanisms. Using this multidisciplinary perspective, Was aims to uncover insights into fairness that are rarely considered in AI work rooted in a single field. The overall work aims to substantiate and demonstrate a deceptively simple conclusion: to create fairer AI, we must ensure we understand fairness (at least) as much as we understand AI.